AI Explainability for Generative AI Chatbots
.webp)
What is explainability in Generative AI?
Explainability in Generative AI refers to understanding and articulating how and why generative AI models produce their outputs. Generative AI models are very effective at producing high-quality new content, such as text, images, or video. Still, it is usually unknown or hard to explain why they did what they did. For example, if I ask a model to write me a short story – it can choose to write a thriller, a romance or a children’s story. Explainability is the ability to explain why it chose one over the other.
Modern Generative AI applications are built upon Retrieval Augmented Generation (RAG) architecture, which retrieves content first (typically from a Vector Data Store) and then uses the content to generate new content using a Large Language Model (LLM). RAG Architecture introduces additional complexity in explaining how a response is generated. In addition to the LLMs, the responses are now affected by decisions across the RAG value chain – retrieval, prioritization, filtering, fusion, etc., which can all impact the result—making it even more difficult to understand why a particular response was generated.
Why is explainability important for Generative AI applications like chatbots?
Explainability is at the heart of ensuring a GenAI application is accurate, trustworthy, fair and consistent. For example, suppose we use an AI application to approve a mortgage application – we need to understand WHY a loan application was rejected or approved. In that case, it should be for the right reasons like your credit score and not for random reasons like what kind of music you listen to). Put another way – if we can’t understand why AI does what it does, we cannot give it control over essential tasks or even trust it to do simple tasks. Not understanding the rationale of an AI system can lead to seriously negative financial and legal consequences and may even have life-and-death implications (autonomous driving, anyone!).
Whether consumer or enterprise, explainability is mission-critical when it comes to chatbots. For example, if an employee has a question about a policy, we want to ensure it is answered based on the correct documents and is relevant to the user's profile. The only way to ensure that this happens consistently is to make sure we can pull apart the curtain and see the inner workings of why the bot is answering or responding the way it does.
Rezolve.ai’s approach to explainability
There are many technical approaches to delivering explainability in the industry. Some of these include:
- Feature Importance and Attribution Methods
- Saliency Maps and Visualizations
- Attention Mechanism Visualization
- Counterfactual Explanations
- Intrinsic Interpretability
- Transparency and Documentation Tools
- Interactive and User-Centric Approaches
Rezolve.ai has taken a hybrid approach to explainability. It is designed from the ground up to be super easy to follow by even a non-technical user (e.g., a copywriter). The system uses a step-by-step decision tree visualization to walk the user through all the decision steps, from retrieving content to generating a response.
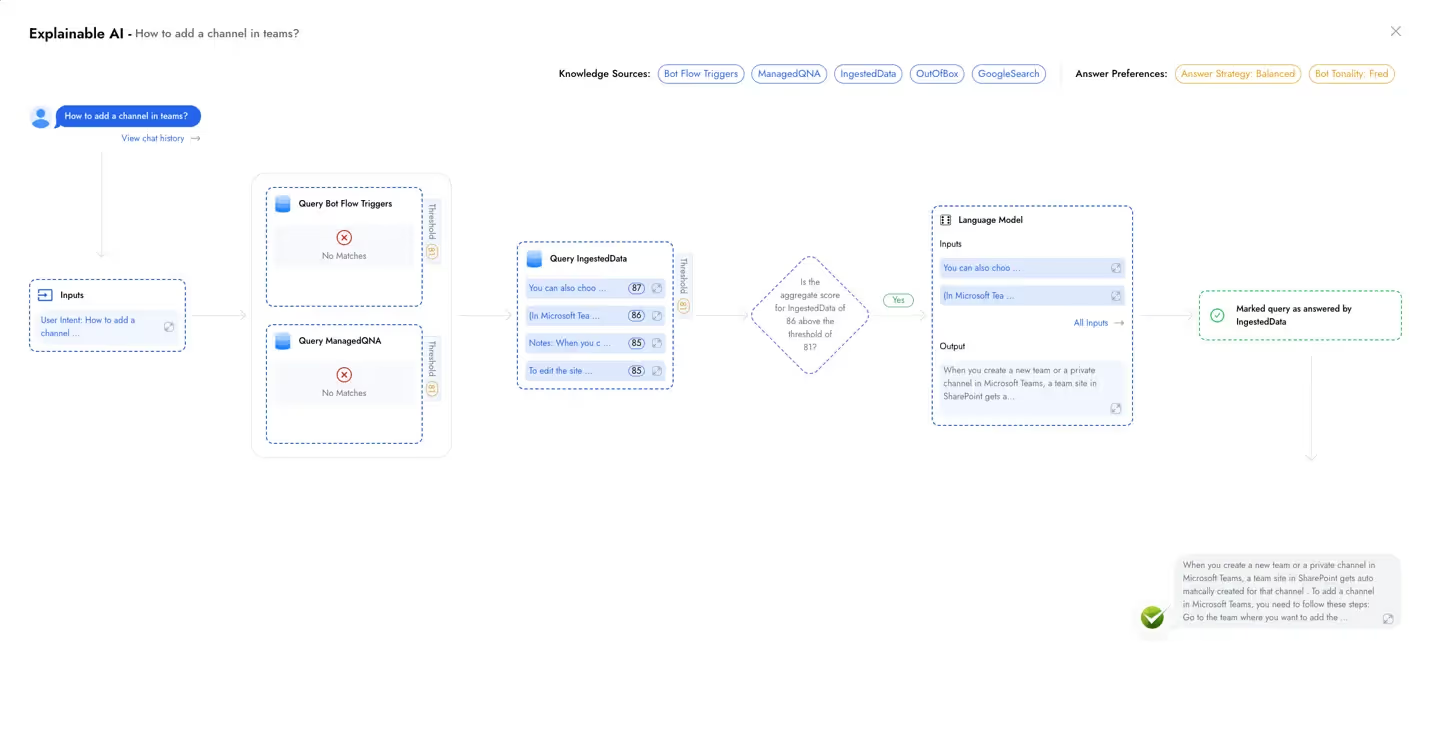
The system is organized like a flow chart and shows the users the content, rules and decisions at each step. For example, if the user asks, "How do I add a Channel in Teams?” The system will follow a set of steps:
- Should it trigger a workflow?
- Does it match a scripted answer (for when you need a precise response)
- What content did it find and retrieve
- How was the content filtered, ranked and prioritized
- Which LLM was used to generate a response
- What was the input and out of the LLM
- What final response did the user see
- How did the user rate the response
All of this is visualized for an admin or power user to see and easily understand, which was the core design principle. Take a difficult idea of explainability and make it accessible to all admins and power users.
So, what are the positive impacts of having good explainability in an AI product?
AI explainability can have significant positive impacts on the platform, including:
- Builds Trust and Transparency: Explainability helps users understand how AI systems make decisions, fostering trust by making the process transparent and comprehensible. This transparency is essential for user adoption and continued good user experience.
- Bot Debugging and Improvement: By understanding how the chatbot responds the way it does, system admins can quickly root cause incorrect or inaccurate responses and adjust as needed.
- Ensuring compliance and fairness: Explainability allows organizations to ensure that there is always a consistent reason why hot bots respond. This ensures fairness and lack of bias across the user population.
- Identify knowledge gaps: The system allows admins to identify knowledge gaps and inconsistent, conflicting, ambiguous or inaccurate content inside their organization by providing appropriate explanations.
- More control and feedback from users: User feedback and input can be an important tool for understanding what is working and why or why not. This can make explainability very actionable for improving user experience and, eventually, adoption and ROI.
Is explainability the same as Data Loss Prevention (DLP) for AI?
While both are important in the context of AI, they address different challenges and serve different functions. Explainability focuses on understanding the why behind AI decisions, whereas DLP focuses on protecting the data. The table below provides a quick overview of this:
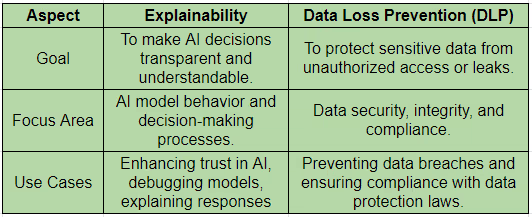
Closing Note
Generative AI is one of the most transformational technologies of the current times. Understanding the why behind what it does using explainability is critical to building user trust. Explainability is the bedrock of building and operating a high ROI AI platform, as good quality responses from a well-understood system drive adoption and usage. The key to explaining explainability well is to make it simple and easy to relate so it is accessible and used widely by users.
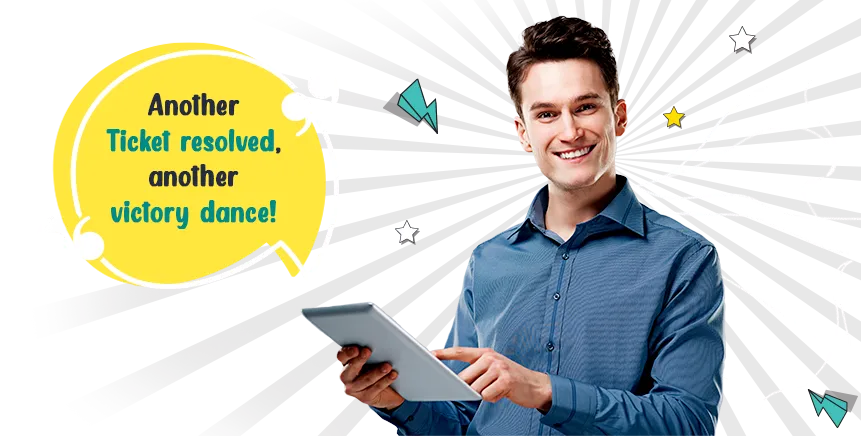
.webp)